[해외 DS] 반세기 만에 찾은 '아인슈타인 타일' ①, 아마추어 수학자의 놀라운 직관
입력
수정
50년 묵은 난제, '아인슈타인 타일' 해결 데이비드 스미스, '모자' 타일 발견해 모노타일 발견으로 타일 이론에 새로운 지평 열려
[해외DS]는 해외 유수의 데이터 사이언스 전문지들에서 전하는 업계 전문가들의 의견을 담았습니다. 저희 데이터 사이언스 경영 연구소 (GIAI R&D Korea)에서 영어 원문 공개 조건으로 콘텐츠 제휴가 진행 중입니다.

2022년 11월 캐나다 워털루대의 크레이그 카플란(Craig S. Kaplan) 교수는 어떤 도형을 봐달라는 이메일을 받았다. 이 이메일 안에는 많은 사람들이 존재할 수 없다고 생각했던 '아인슈타인 타일'로 보이는 '모자' 도형이 그려져 있었다. 타일링(평면을 덮기 위해 도형을 배열하는 다양한 방법)에 관심 있는 '도형 애호가' 데이비드 스미스(David Smith)가 보낸 이메일인데, 그는 영국 요크셔에 있는 자택에서 여가 시간에 기하학 실험을 즐겨 하는 아마추어 수학자다. 그런 그가 반세기 동안 전이 없던 이 문제를 풀어냈다. 반복된 패턴 없이 단 하나의 모양으로 평면을 무한대로 메울 수 있는 '비주기적 모노타일(aperiodic monotile)'을 발견한 것이다.
카플란 교수는 스미스와 정기적으로 연락을 주고받으며 카플란 교수가 만든 프로그램에서 모자 타일로 평면이 무한대로 채워질 수 있는지 확인했다. 2023년에는 엄밀한 수학적 증명을 위해 타일링 이론 분야에서 잘 알려진 수학자 차임 굿맨-슈트라우스(Chaim Goodman-Strauss)와 소프트웨어 개발자 조셉 사무엘 마이어스(Joseph Samuel Myers)에게 추가로 연락을 취했다. 굿맨-슈트라우스 수학자와 마이어스가 모자 모양의 타일이 비주기적 모노타일임을 증명하는 동안 스미스로부터 새로운 메일이 도착했다. 메일에는 "또 다른 아인슈타인 타일을 찾은 것 같다"고 적혀있었다. 스미스의 모자는 시작에 불과했다. 이 모자는 '거북이', '유령', 그리고 예상했던 것보다 더 많은 통찰력을 제공하는 다른 수학적 경이로움으로 이어졌다.
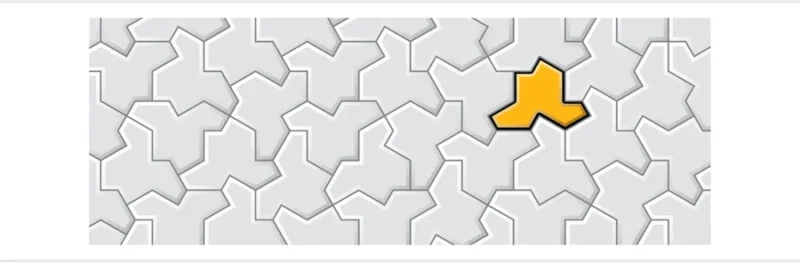
평면을 무한히 채우기 위해 필요한 두 가지 속성
수학자들이 본격적으로 타일을 연구하기 시작한 것은 20세기부터다. 이른바 평면의 타일링은 평면을 빈틈없이, 겹치지 않고 덮는 도형의 무한한 집합을 말한다. 여기서는 타일링에 포함된 무한히 많은 타일이 유한한 개수의 서로 다른 모양을 한 경우에 초점을 맞춘다. 무한한 테이블 위에 타일 도형을 잘라내어 테이블의 모든 부분이 한 겹의 종이로 덮이도록 하는 것이다. 반사(종이를 뒤집는 것), 회전(그 자리에서 돌리는 것), 평행이동(돌리지 않고 도형을 미는 것)을 조합하여 타일을 평면 위에 채워나갈 수 있다. 그 결과 도형의 집합은 타일링을 '인정'하게 되고, 더 일반적으로는 도형이 평면을 타일링할 수 있다고 표현한다.
모든 도형 집합이 타일링을 허용하는 것은 아니다. 정사각형은 모노타일(하나의 집합)로서 평면을 타일링 하지만, 정오각형은 그 자체로는 평면을 타일링할 수 없다. 마찬가지로 정팔각형도 스스로 평면을 타일링할 수 없지만, 정팔각형과 정사각형으로 구성된 집합은 평면을 타일링 할 수 있다.
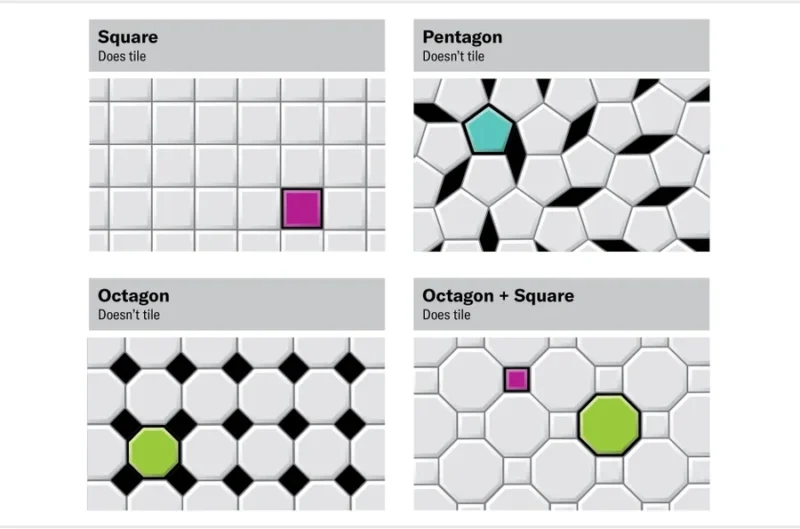
그렇다면 주어진 도형 집합이 평면을 타일링하는지 어떻게 확인할 수 있을까. 이 질문에 답할 수 있는 알고리즘은 존재하지 않으며, 실제로 존재할 수도 없다. 이는 이론 컴퓨터 과학에서 '결정 불가능'으로 알려져 있다. 하지만 다른 방법을 통해 타일링을 증명할 수 있는데, 스미스의 모자가 등장하기 전에는 항상 두 가지 방식 중 하나로 작동했다.
첫 번째 속성은 도형을 그 자체의 복사본으로 완전히 둘러싸는 것을 시도해 보는 것이다. 그럴 수 없다면 도형은 타일링을 허용하지 않는 것이 확실하다. 하지만 한 층으로 에워싸는 것으로 타일링을 담보하지 못한다. 하나 이상의 동심층을 허용하는 기만적인 '비타일러'가 있기 때문이다. 1968년 수학자 하인리히 히쉬(Heinrich Heesch)는 한 번은 둘러싸일 수 있지만 두 번은 둘러싸일 수 없는 도형을 보여주며 비타일러 주위에 만들 수 있는 동심원의 수에 상한이 있는지 물었고, 이 수치는 도형의 '히쉬수'(Heesch number)로 알려져 있다. 현재 가장 높은 히쉬수는 6이며, 세르비아 노비사드대학의 보얀 바시치가 2020년에 발견한 히쉬 수 6은 매우 화려한 다각형이다.
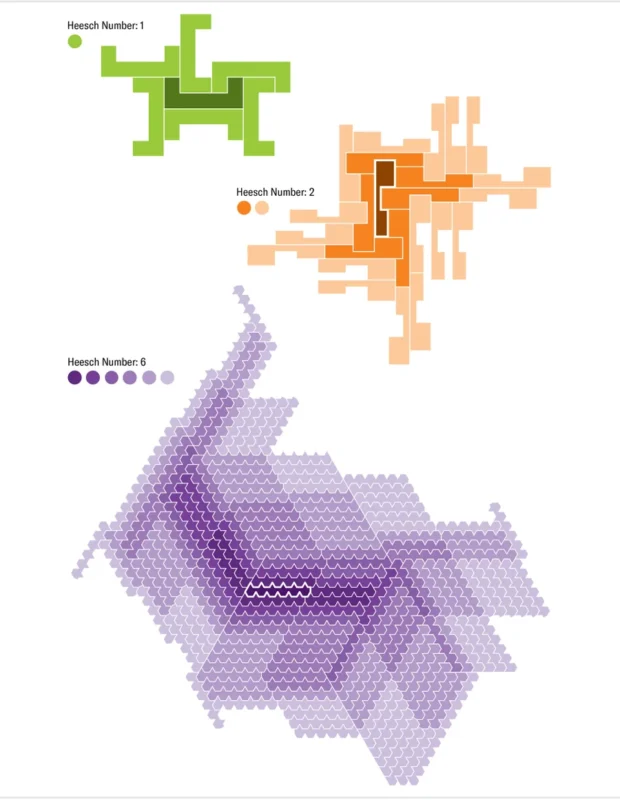
두 번째 속성은 도형이 주기적으로 평면을 타일링한다는 특성을 발견하는 것이다. 주기적 타일링에서는 타일의 배열이 무한한 평행 사변형 격자 위에 규칙적인 패턴으로 반복된다. 즉 병진 단위(translational unit)라고 하는 유한한 타일 클러스터를 식별해서 해당 병진 단위의 복사본이 병진 이동을 통해 평면을 무한하게 덮을 수 있는지 확인하는 것이다. 히쉬 수와 마찬가지로, 도형이 평면을 타일링하기 위해 필요한 최소 병진 단위의 하한이 있는지는 아무도 모른다. 마이어스가 발견한 최소 병진 단위는 10개의 타일이었다.
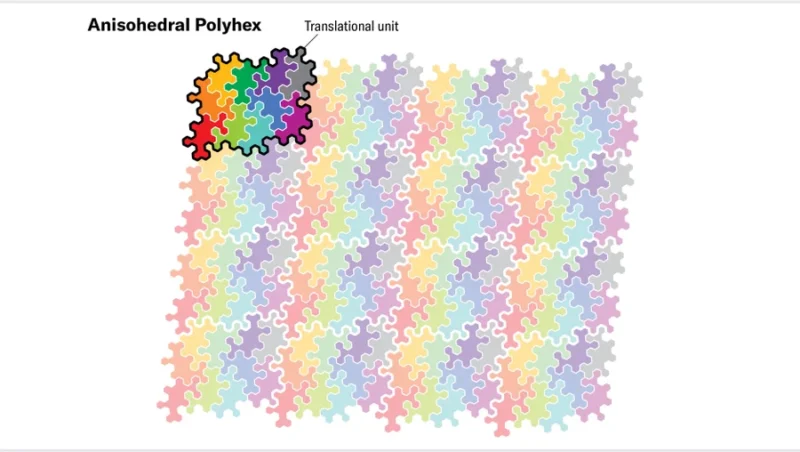
스미스에게 모자 타일이 특별해 보였던 이유는 모자 도형이 위에서 언급한 두 가지 속성 중 어느 것도 따르지 않고 평면을 타일링할 수 있다고 느꼈기 때문이다. 그는 모자의 병진 단위가 몇 개로 이뤄져 있는지 알아낼 수 없었지만, 모자의 히쉬수는 계속 증가하는 것을 발견했다. 물론 모자가 히쉬수가 높은 비타일러이거나 병진 단위가 큰 주기적 모노타일일 수도 있지만, 스미스는 그런 경우가 드물다는 것을 알고 있었다. 그는 한 가지 가능성(비주기적 모노타일, 또는 아인슈타인 타일)이 남아 있다는 것을 알고 있었기 때문에 카플란 교수에게 연락을 취했다.
비주기적 모노타일을 찾기 위한 여정
약 60년 전 수학자들은 주기적으로 반복되지 않고 평면을 타일링할 수 있는 도형 집합, 즉 병진 단위 없이 임의로 큰 주기적 타일링을 형성할 수 없는 도형 집합이 있는지 궁금해하기 시작했다. 이러한 집합을 강한 비주기성(aperiodicity)이라고 하는데, 일반 비주기성(nonperiodicity)에서 임의로 큰 주기적 타일링이 없다는 조건이 추가된 속성이다. 예를 들어 평범한 2×1 직사각형을 포함한 많은 도형에서 약간의 배치 변형으로 주기성과 비주기성을 모두 허용하는데, 강한 비주기성은 어떤 주기성도 허용하지 않는다.
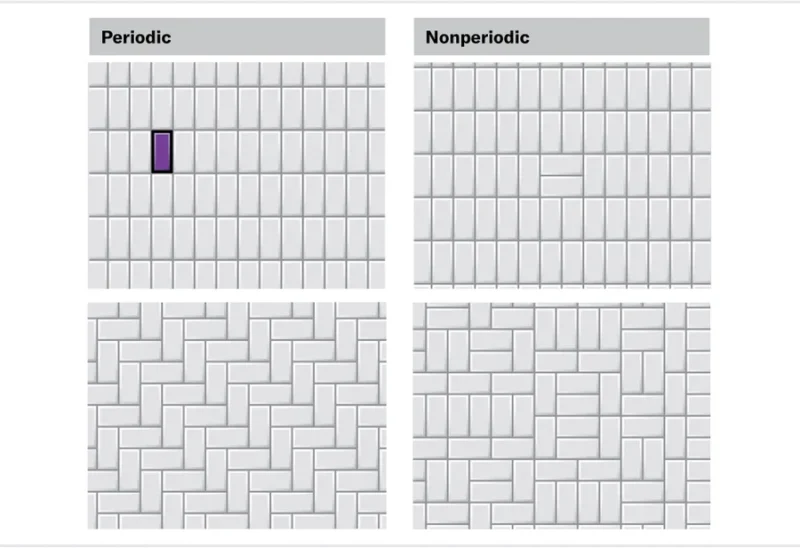
강한 비주기성은 1960년대 초 하버드대학교의 수학과 교수로 재직 중이던 하오 왕(Hao Wang)이 처음 설명한 개념이다. 그는 현재 우리가 '왕 타일'이라고 부르는 정사각형 타일, 즉 가장자리에 라벨이나 색상이 있는 정사각형 타일을 연구하고 있었는데, 타일 집합이 주어졌을 때 위쪽과 아래쪽 가장자리의 레이블 순서가 같고 왼쪽과 오른쪽 가장자리도 일치하는 직사각형을 찾을 수 있다면, 그 직사각형은 병진 단위이므로 그 집합이 평면을 타일링한다는 것을 관찰했다. 그는 반대로 왕 타일 집합이 평면을 무한히 타일링할 수 있으면 그러한 직사각형을 만들 수 있어야 한다고 추측했다. 따라서 그는 왕 타일이 결코 강한 비주기성을 가질 수 없다고 주장했다.
당시 타일링에 대해 알려진 바에 따르면 왕의 추측은 상당히 합리적이었다. 그러나 몇 년 후 왕의 제자인 로버트 버거(Robert Berger)는 이 연구를 바탕으로 20,426개의 왕 타일로 구성된 최초의 비주기적 타일 세트를 구성해 냈다. 버거는 더 작은 비주기적 집합을 발견하기 위해 집합의 크기가 얼마나 작을 수 있는지에 대한 거부할 수 없는 수학적 탐구을 시작했다. 1971년 미국 버클리 캘리포니아대학의 라파엘 M. 로빈슨(Raphael M. Robinson)은 6개의 변형된 정사각형 집합을 찾아냈다.
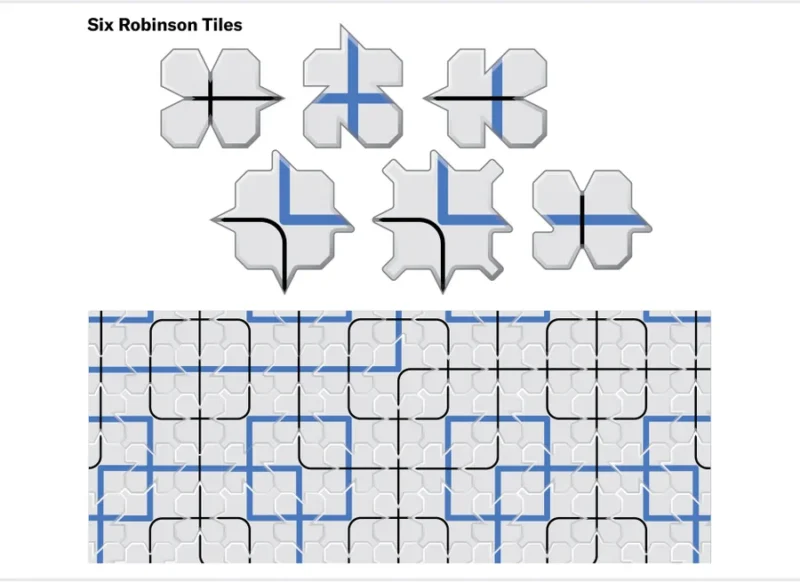
그리고 1973년 옥스퍼드대의 수학자 로저 펜로즈(Roger Penrose)는 '연'(kite)과 '다트'(dart)라는 단 두 개의 타일로 이뤄진 결과를 선보였다.
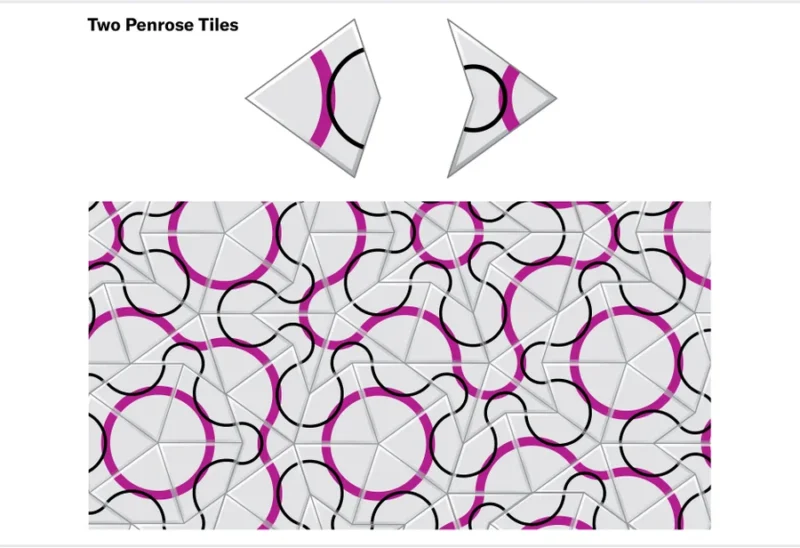
펜로즈의 연구로 비주기적 모노타일(aperiodic monotile)이라는 결승선으로부터 한 걸음만 남겨두게 됐다. 비주기적 모노타일은 '하나의 돌'이라는 뜻의 독일어 '아인슈타인'에서 유래한 '아인슈타인 타일'이라고도 불린다. 그리고 비주기적 모노타일이 존재하는지에 대한 질문은 아인슈타인 문제라고 불린다.
펜로즈 이후 거의 50년 동안 진전이 없었다. 굿맨-슈트라우스가 발견한 것을 포함해 몇 가지 다른 듀얼 집합이 발견됐을 뿐이고, 일부 수학자들이 제안한 단일 타일은 타일 게임 규칙을 수정해야 했다. 예를 들어 소콜라-테일러 타일(Socolar-Taylor Tile)은 비주기적으로 배열되도록 하려면 육각형을 3차원으로 돌출시키거나 단절된 조각으로 쪼개는 등의 수정을 거쳐야 했다.
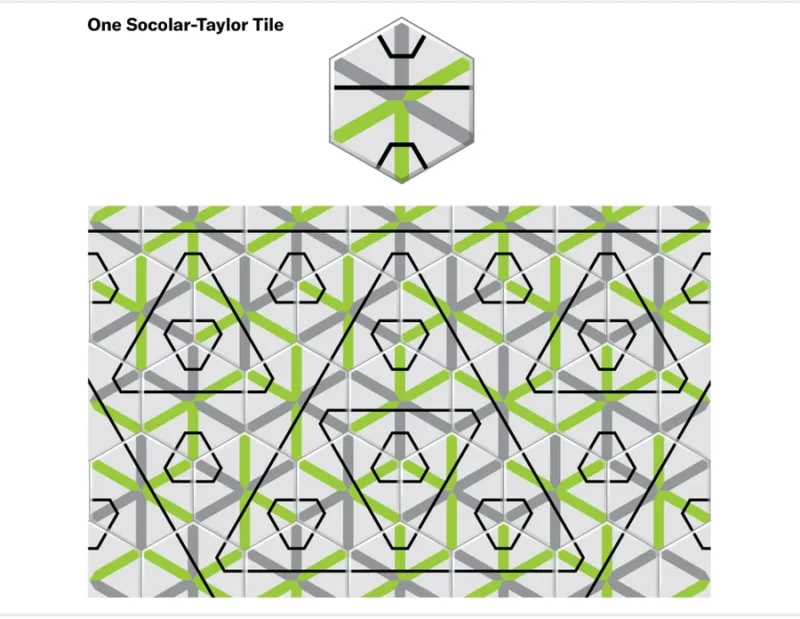
그럼에도 많은 이들이 아인슈타인 문제에 매료됐던 이유 중 하나는 비주기적 모노타일의 존재를 지지하거나 반대하는 명확한 증거가 보이지 않았기 때문이다. 일부 수학자들은 비주기적 모노타일이 존재할 수 없다고 단념했지만, 희망을 가진 이들은 존재 증명이 존재하지 않는다는 증명보다 더 설득력이 있을 것으로 생각하며 묵묵히 연구를 이어 나갔다. 스미스는 결국 모노타일을 발견했고, 이는 오랜 침체의 끝을 알리듯 창발의 연속으로 이어졌다.
[해외 DS] 반세기 만에 찾은 '아인슈타인 타일' ②, 아마추어 수학자의 샘솟는 아이디어으로 이어집니다.
Inside Mathematicians’ Search for the Mysterious ‘Einstein Tile’
The quest for the einstein tile—a shape never seen before in mathematics—turned up even more discoveries than mathematicians counted on
In November 2022 a colleague of mine casually asked what I was working on. My dazed answer reflected the swirl of ideas that was consuming all my mental energy at the time: “Actually, I think the solution to a major open problem just fell into my lap.” A week before, I had received an e-mail asking me to look at a shape. That was the first time I saw “the hat,” an unassuming polygon that turned out to be the culmination of a decades-long mathematical quest.
The e-mail came from David Smith, someone I knew from a small mailing list of people interested in tilings—different ways to arrange shapes to cover a flat surface. Smith isn't a mathematician; he is a self-professed “shape hobbyist” who experiments with geometry in his spare time from his home in Yorkshire, England. After Smith sent me the hat shape he'd been playing with, we began corresponding regularly, spending the rest of 2022 studying the hat and its properties. In 2023 we reached out to two additional researchers, mathematician Chaim Goodman-Strauss and software developer Joseph Samuel Myers, both also members of the mailing list and well known in the larger world of tiling theory. The four of us continued to study the hat and, in what felt like record time, succeeded in proving that the shape was a long-sought object that many assumed couldn't exist: an aperiodic monotile, also known as an einstein tile.
As it turns out, Smith's hat was just the beginning of a sequence of revelations. As we explored the new landscape of ideas revealed by this shape, we were surprised multiple times by additional discoveries that further deepened our understanding of tiling theory. Soon the hat led to “turtles,” “spectres,” and other wonders that yielded more insights than we could have expected at the outset.
Tiles have fascinated humans since ancient times, but mathematicians began studying them in earnest in the 20th century. A so-called tiling of the plane is an infinite collection of shapes that cover a flat surface with no gaps and no overlaps. I will focus on cases where the infinitely many tiles in a tiling come in a finite number of distinct shapes. Imagine a handful of templates that can be used to cut copies of the shapes out of an unlimited supply of paper. Our goal is to arrange cutouts on an infinite tabletop so that every bit of table is covered by exactly one layer of paper. We can move each cutout into position through some combination of reflection (flipping the paper over), rotation (turning it in place) and translation (sliding the shape around without turning it). If we achieve our goal of constructing a tiling, we say that the set of shapes “admits” the tiling and, more generally, that the shapes tile the plane.
Not all sets of shapes admit tilings. A square yields a tiling resembling graph paper, among other patterns, and is therefore a monotile: it tiles the plane on its own (as a set of one). A regular pentagon, in contrast, cannot tile the plane by itself. Neither can a regular octagon, although a two-element set consisting of an octagon and a square does tile.
How can we determine whether a given set of shapes tiles the plane? There's no algorithm we can use to answer this question, and in fact none could exist—the problem is what's known in theoretical computer science as “undecidable.” Nevertheless, we can study individual sets and attempt to build tilings through trial and error or other methods. Along the way we often encounter fascinating examples of how local interactions (the different ways two tiles can sit side-by-side) influence global behavior (the large-scale structure of the tiling out to infinity in every direction).
There are multiple ways to figure out whether a single shape can tile the plane. Some people, such as Smith, will even cut out physical paper copies of a shape using a computer-controlled cutting tool and play with them on actual (regrettably finite) tabletops, recruiting the immediacy of touch to augment visual intuition. In the hands of a skilled explorer like Smith, a shape will disclose its tiling secrets in short order. And in the pre-hat era, a shape would invariably behave in one of two ways.
The first possibility is that the shape will not tile the plane. As a quick test, we might try to surround it completely by copies of itself; if we can't, then the shape certainly does not admit any tilings. For instance, the regular pentagon is unsurroundable, which immediately outs it as a nontiler. But although surroundability provides evidence of tilability, it is not firm proof: there are deceptive nontilers that can be completely surrounded by one or more concentric layers of copies before getting irretrievably stuck. In 1968 mathematician Heinrich Heesch exhibited a shape that could be surrounded once but not twice and asked whether there was an upper limit to the number of concentric rings one might build around a nontiler, a quantity now known as a shape's “Heesch number.” The current record holder is a particularly ornery polygon with a Heesch number of six, discovered in 2020 by Bojan Bašić of the University of Novi Sad in Serbia.
The second possibility is that the shape tiles the plane periodically. In a periodic tiling, the arrangement of tiles repeats in a regular pattern determined by an infinite grid of parallelograms. We can describe a periodic tiling using three pieces of information: a finite cluster of tiles called a translational unit and two line segments that define the sides of a parallelogram in the grid. We can slide a copy of the translational unit out to every vertex in the grid, without rotating or reflecting it, and these copies will interlock to complete a tiling. This method offers a quick test of a shape's ability to tile: we assemble candidate translational units and then see whether any of them covers the plane by repeating in a regular grid. As with Heesch numbers, no one knows whether there is any bound on the smallest translational unit a shape might require before it can be repeated to tile the plane. Myers discovered the current record holder, a shape whose simplest translational unit contains 10 tiles.
When Smith began experimenting with the hat, what caught his eye was that it refused to conform to either of these options. The hat did not obviously tile the plane: he couldn't find a way to build a translational unit of any size. But it did not obviously fail to tile the plane, either: with effort, he could surround a hat with multiple layers of copies without getting stuck. It was conceivable that the hat might be a nontiler with a high Heesch number or a periodic monotile with a large translational unit, but Smith knew that such cases were rare. He reached out to me because he also knew that there was one other possibility, one so extraordinary that it demanded to be considered in full.
About 60 years ago mathematicians started wondering whether there were sets of shapes that could only tile the plane without ever repeating periodically—that is, that someone could assemble copies into arbitrarily large patches without ever encountering a translational unit. Such a set is called aperiodic. Crucially, aperiodicity is a much stronger property than nonperiodicity. Lots of shapes, including a humble 2 × 1 rectangle, can admit tilings that are periodic as well as tilings that aren't periodic. Aperiodic sets have no possible periodic tilings.
The notion of aperiodicity was first articulated by Hao Wang in the early 1960s, while he was a math professor at Harvard University. He was studying what we now call Wang tiles: square tiles with symbolic labels or colors on their edges that must be positioned so that neighboring squares have the same markings on their adjoining edges. (These labels are a convenient shorthand for equivalent rules that can be expressed geometrically.) Wang observed that if, given a set of tiles, one can find a rectangle whose top and bottom edges have the same sequence of labels and whose left and right edges also match, then that rectangle is a translational unit, and hence the set tiles the plane. He then conjectured the converse: that if a set of Wang tiles admits a tiling of the plane, then it must be possible to build such a rectangle. In other words, he claimed that Wang tiles can never be aperiodic.
Based on what was known about tilings at the time, Wang's conjecture was quite reasonable. Building on this work a few years later, however, Wang's student Robert Berger disproved the conjecture by constructing the first aperiodic tile set, a sprawling system of 20,426 Wang tiles. In passing, Berger speculated that it should be possible to construct smaller aperiodic sets, inaugurating an irresistible mathematical quest to see how small a set could be. By 1971 Raphael M. Robinson of the University of California, Berkeley, had gotten down to a set of six modified squares.
Then, in 1973, University of Oxford mathematician Roger Penrose achieved a stunning breakthrough with a set of just two tiles: the “kite” and the “dart.”
Penrose's work left us one step short of an obvious finish line: an aperiodic monotile, a single shape that admits only nonperiodic tilings. Such a shape is also sometimes called an “einstein,” from the German “ein stein,” meaning “one stone.” (It's a pun on the name “Einstein” but otherwise has no connection to the famous Albert.) The question of whether an aperiodic monotile exists has been called the einstein problem.
After Penrose, progress stalled for nearly 50 years. A few other sets of size two were discovered, including one by Goodman-Strauss. Some mathematicians proposed single-shape solutions, but these inevitably required small amendments to the rules of the game. For example, the Socolar-Taylor tile is a modified regular hexagon that tiles aperiodically. The catch is that for copies of this hexagon to conspire to force all tilings to be aperiodic, nonadjacent tiles must come to an agreement about their relative orientations. There is no way to bake this restriction into the outline of the tile without introducing a trick, such as extruding the hexagon into three dimensions or breaking it into disconnected pieces.
Even when a problem in mathematics is unsolved, there is often a broad consensus among mathematicians about its likely answer. For example, Goldbach's conjecture states that every even number greater than two is the sum of two odd primes. This conjecture is unproven, but the evidence we have overwhelmingly suggests that it's correct. One reason I was always fascinated by the einstein problem is that I did not see clear evidence for or against it (apart from the grim reality of a 50-year dry spell). Some mathematicians were resigned to the impossibility of aperiodic monotiles, but I was open to either outcome. If nothing else, I suspected that an existence proof would be more tractable than a nonexistence proof. The former was likely to be an argument about the properties of a specific shape, but the latter would necessarily be a statement about all shapes. As we now know, in this instance there is some justice in the universe.