입력
수정
[해외DS]는 해외 유수의 데이터 사이언스 전문지들에서 전하는 업계 전문가들의 의견을 담았습니다. 저희 데이터 사이언스 경영 연구소 (GIAI R&D Korea)에서 영어 원문 공개 조건으로 콘텐츠 제휴가 진행 중입니다.
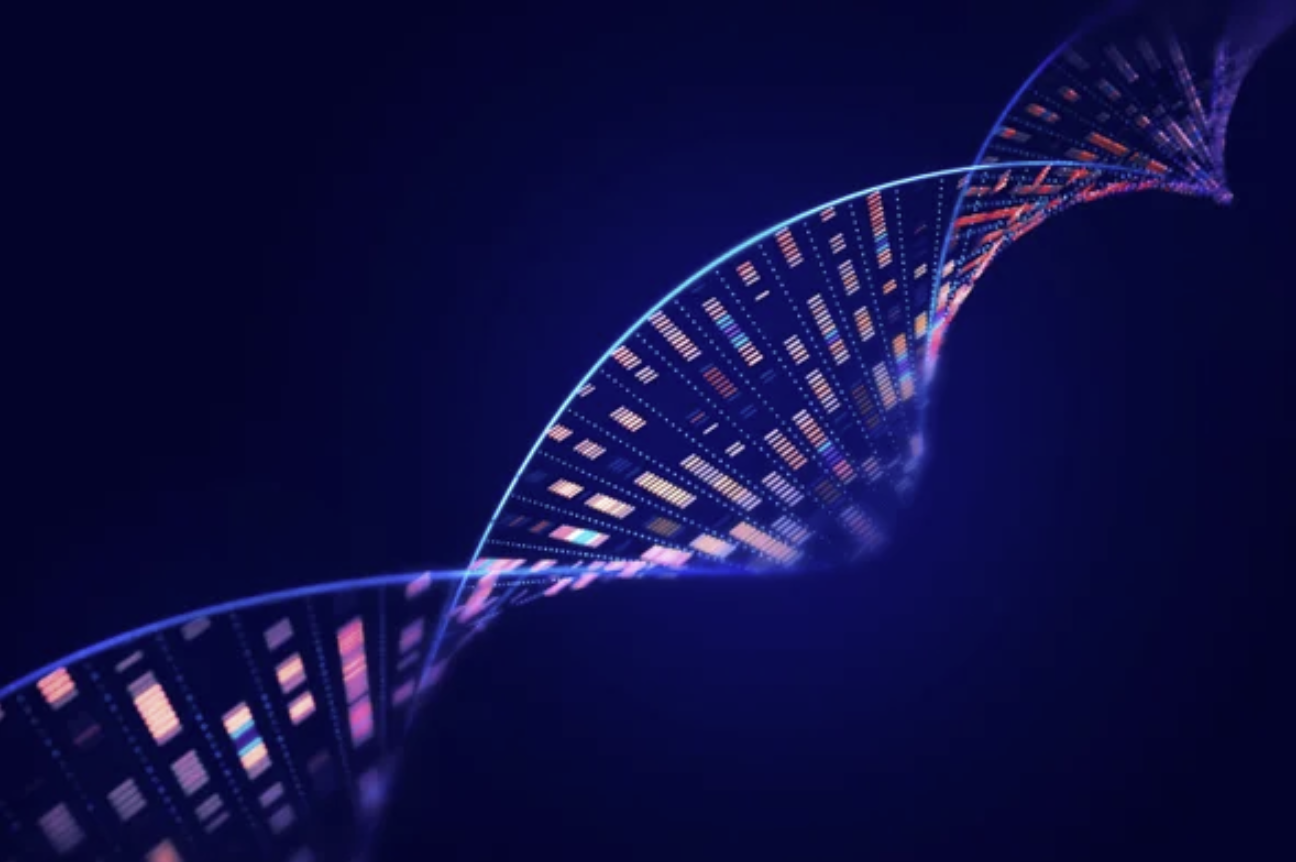
9월 19일(현지 시각) 사이언스(Science)에 실린 논문에서 구글 딥마인드의 AlphaFold 네트워크에 기반한 새로운 도구 AlphaMissense가 건강 상태를 악화할 가능성이 있는 단백질의 돌연변이를 정확하게 예측할 수 있다고 전해졌다. AlphaMissense는 의사가 질병의 원인을 찾기 위해 사람의 게놈을 '해석'하는 데 도움을 주기 위해 개발 중인 많은 기술 중 하나이며, 실제로 사용되기 전에 철저한 테스트를 거쳐야 한다고 해당 연구팀이 강조했다.
낭포성 섬유증과 낫 모양 적혈구 빈혈증같이 질환을 직접적으로 유발하는 많은 유전적 돌연변이는 단백질의 아미노산 배열을 변경하는 경향이 있다. 전문가들에 따르면 현재까지 관찰된 미센스 돌연변이는 수백만 개에 불과하며, 이 돌연변이는 7천만 개 이상의 변이가 가능하다고 알려져 있다. 설상가상으로 질병과 결정적으로 연관된 돌연변이는 극히 일부에 지나지 않기 때문에 연구자와 의사가 이전에 본 적이 없는 미센스 돌연변이를 발견했을 때, 이를 어떻게 해석해야 할지 알기 어려운 상황에 직면한다. 따라서 연구자들은 변이가 질병을 일으킬 가능성이 있는지 예측할 수 있는 수십 가지의 다양한 계산 도구를 개발했다. 그 중 AlphaMissense는 기존 접근 방식을 통합하고 기계학습을 통해 점점 더 많은 문제를 해결하기 위해 개발됐다.
AlphaFold와 ChatGPT 장점 살려 돌연변이 발생 위치 예상
AlphaMissense는 아미노산 배열로부터 단백질 구조를 예측하는 AlphaFold의 구조에 대한 직관을 사용하여 단백질 내에서 질병을 유발하는 돌연변이가 발생할 위치를 식별한다고 딥마인드 연구 부사장이자 연구 저자인 푸미트 콜리(Pushmeet Kohli)가 언론 브리핑에서 밝혔다. 또한 단어 대신 수백만 개의 단백질 배열을 학습한 ChatGPT와 같은 단백질 언어 모델이 포함되어 있다. 이 모델은 어떤 서열이 그럴듯하고 어떤 서열이 그렇지 않은지 학습했기 때문에 변종 예측에 유용하다.
딥마인드의 네트워크는 수천 개의 돌연변이의 영향을 한 번에 측정하는 실험에서 문제 변이를 발견하는 데도 효과적인 것으로 나타났다. 연구진은 또한 AlphaMissense를 사용하여 인간 게놈에서 가능한 모든 미센스 돌연변이의 카탈로그를 작성하여 57%는 유해하지 않을 가능성이 높고 32%는 질병을 유발할 수 있다고 판단했다.
사람의 생명과 맞닿기 때문에 엄밀한 검증 필요
스톡홀름 대학의 계산 생물학자인 아르네 엘로프손(Arne Elofsson)은 AlphaMissense은 돌연변이의 영향을 예측하는 기존 도구보다 발전했지만 "엄청난 도약은 아니다"라고 꼬집었다. 영국 에든버러에 있는 MRC 인간 유전학 유닛의 계산 생물학자 조셉 마쉬(Joseph Marsh)도 계산 생물학의 새로운 시대를 연 AlphaFold만큼의 영향력은 없을 것이라고 동의했다. 마쉬는 현재 컴퓨터 예측은 유전 질환을 진단하는 데 최소한의 역할만 하고 있으며, 의사 단체의 권고에 따르면 이러한 도구는 돌연변이와 질병의 연관성을 뒷받침하는 증거만 제공해야 한다고 설명했다.
조지아주 애틀랜타에 있는 에모리 대학교의 생물정보학자인 야나 브롬버그(Yana Bromberg)는 AlphaMissense같은 도구가 실제 세계에 적용되기 전에 엄격하게 평가되어야 한다고 강조했다. 글로벌 인공지능 유전체 분석 경진대회(CAGI)에서 입증된 모델을 사용해야 한다는 입장이다. 의료 분야 특성상 거짓 음성(false negative)에 민감하기 때문에 다른 예측 모델보다 유독 엄격한 잣대를 적용해야 한다는 기조가 깔려있다.
AI Tool Pinpoints Genetic Mutations That Cause Disease
Researchers have adapted the AI network to search for genetic changes linked to ill health
Google DeepMind has wielded its revolutionary protein-structure-prediction AI in the hunt for genetic mutations that cause disease.
A new tool based on the AlphaFold network can accurately predict which mutations in proteins are likely to cause health conditions — a challenge that limits the use of genomics in healthcare.
The AI network — called AlphaMissense — is a step forward, say researchers who are developing similar tools, but not necessarily a sea change. It is one of many techniques in development that aim to help researchers, and ultimately physicians, to ‘interpret’ people’s genomes to find the cause of a disease. But tools such as AlphaMissense — which is described in a 19 September paper in Science — will need to undergo thorough testing before they are used in the clinic.
Many of the genetic mutations that directly cause a condition, such as those responsible for cystic fibrosis and sickle-cell disease, tend to change the amino acid sequence of the protein they encode. But researchers have observed only a few million of these single-letter ‘missense mutations’. Of the more than 70 million possible in the human genome, only a sliver have been conclusively linked to disease, and most seem to have no ill effect on health.
So when researchers and doctors find a missense mutation they’ve never seen before, it can be difficult to know what to make of it. To help interpret such ‘variants of unknown significance,’ researchers have developed dozens of different computational tools that can predict whether a variant is likely to cause disease. AlphaMissense incorporates existing approaches to the problem, which are increasingly being addressed with machine learning.
LOCATING MUTATIONS
The network is based on AlphaFold, which predicts a protein structure from an amino-acid sequence. But instead of determining the structural effects of a mutation — an open challenge in biology — AlphaMissense uses AlphaFold’s ‘intuition’ about structure to identify where disease-causing mutations are likely to occur within a protein, Pushmeet Kohli, DeepMind’s vice-president of Research and a study author, said at a press briefing.
AlphaMissense also incorporates a type of neural network inspired by large language models like ChatGPT that has been trained on millions of protein sequences instead of words, called a protein language model. These have proven adept at predicting protein structures and designing new proteins. They are useful for variant prediction because they have learned which sequences are plausible and which are not, Žiga Avsec, the DeepMind research scientist who co-led the study, told journalists.
DeepMind’s network seems to outperform other computational tools at discerning variants known to cause disease from those that don’t. It also does well at spotting problem variants identified in laboratory experiments that measure the effects of thousands of mutations at once. The researchers also used AlphaMissense to create a catalogue of every possible missense mutation in the human genome, determining that 57% are likely to be benign and that 32% may cause disease.
CLINICAL SUPPORT
AlphaMissense is an advance over existing tools for predicting the effects of mutations, “but not a gigantic leap forward,” says Arne Elofsson, a computational biologist at the University of Stockholm.
Its impact won’t be as significant as AlphaFold, which ushered in a new era in computational biology, agrees Joseph Marsh, a computational biologist at the MRC Human Genetics Unit in Edinburgh, UK. “It’s exciting. It’s probably the best predictor we have right now. But will it be the best predictor in two or three years? There’s a good chance it won’t be.”
Computational predictions currently have a minimal role in diagnosing genetic diseases, says Marsh, and recommendations from physicians’ groups say that these tools should provide only supporting evidence in linking a mutation to a disease. AlphaMissense confidently classified a much larger proportion of missense mutations than have previous methods, says Avsec. “As these models get better than I think people will be more inclined to trust them.”
Yana Bromberg, a bioinformatician at Emory University in Atlanta, Georgia, emphasizes that tools such as AlphaMissense must be rigorously evaluated — using good performance metrics — before ever being applied in the real-world.
For example, an exercise called the Critical Assessment of Genome Interpretation (CAGI) has benchmarked the performance of such prediction methods for years against experimental data that has not yet been released. “It’s my worst nightmare to think of a doctor taking a prediction and running with it, as if it’s a real thing, without evaluation by entities such as CAGI,” Bromberg adds.