์ค์ด๋ ์ํ ์ ํฌ๋งํผ ๋ฎ์์ง ํฌ๋งํด์ง ๋ฌธํฑ, ์ ํ์ํ์ 30๋๋ ๋์์
์ ๋ ฅ
์์
์ถ์ ์ฐ๋ ๋ฐ๋ผ ํน๋ณํด์ง๊ธ ์ฐจ๋ฑ ์ง๊ธ
๊ฒฝ๊ธฐ ๋ถํฉยท์ ๊ตญ ํผ๋์ ํด์ง์ ์ ๋ฒ ๋ณต์ก
์คํ๋ผ์ธ ์ ํฌ ์ค๋ฉฐ ์ธ๋ ฅ ์ถ์ ๋ถ๊ฐํผ
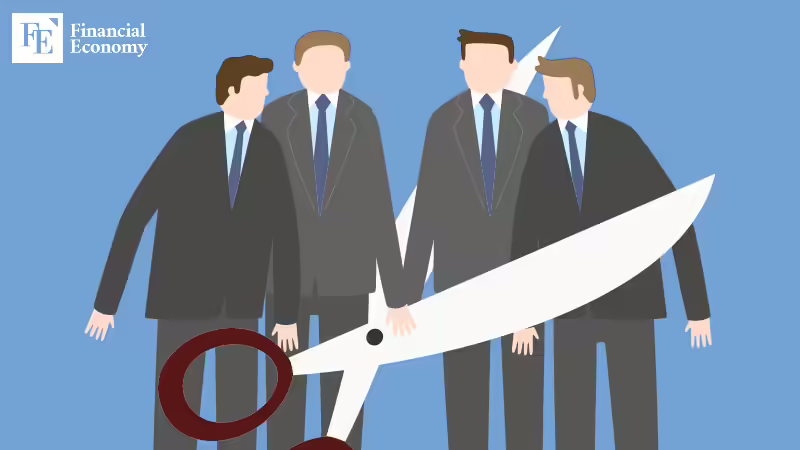
์ ํ์ํ์ด 13์ผ๋ถํฐ ๋ท์๊ฐ ํฌ๋งํด์ง ์ ์ฒญ์ ๋ฐ๋๋ค. ์ง๋ํด ์ฒ์ 30๋ ํ์๊น์ง ์ ์ฒญ ๋์์๋ฅผ ๋ํ ์ ํ์ํ์ ์ฌํด๋ ๊ทธ ๋ฒ์๋ฅผ ์ํญ ํ๋ํ๋ค. ์ํ๊ถ์ ์ฐ๋กํ์ฌ์ธ ํฌ๋งํด์ง ์์ฆ์ด ๋๋ํ ๊ฐ์ด๋ฐ, ์ ๊ณ ์ข ์ฌ์๋ค์ ์ต์ ์ ํ์ด๋ฐ๊ณผ ์กฐ๊ฑด ๋ฑ์ ์ ์ธ์งํ๋ ๋ชจ์ต์ด๋ค.
1986๋ ์๋ ์ง ์ผ๋ค
13์ผ ๊ธ์ต๊ถ์ ๋ฐ๋ฅด๋ฉด ์ ํ์ํ์ ์ด๋ ๋ถํฐ ์ค๋ 17์ผ๊น์ง ํฌ๋งํด์ง ์ ์ฒญ์ ๋ฐ๋๋ค. ํฌ๋งํด์ง ์ ์ฒญ ๋์์ โฒ๋ถ๋ถ์ฅยท๋ถ์ง์ ์ฅ(Ma) ์ด์ ์ง์ ์ค ๊ทผ์ 15๋ ์ด์, 1966๋ ์ดํ ์ถ์ ์ง์ โฒ4๊ธ ์ดํ ์ง์ ์ค ๊ทผ์ 15๋ ์ด์, 1972๋ ์ด์ ์ถ์ ์ง์ โฒ๋ฆฌํ ์ผ์๋น์ค ์ง์ ์ค ๊ทผ์ 7.5๋ ์ด์, 1986๋ ์ด์ ์ถ์ ์ง์์ด๋ค. ํน๋ณํด์ง๊ธ์ผ๋ก๋ ์ถ์ ์ฐ๋์ ๋ฐ๋ผ ์ํ๊ท ์๊ธ์ 7~31๊ฐ์๋ถ ์๊ธ์ด ์ง๊ธ๋๋ค. ์ด๋ ์ง๋ํด ํฌ๋งํด์ง๊ณผ ๋์ผํ ์์ค์ด๋ค.
์ ํ์ํ์ ์ง๋ํด ๋ ์ฐจ๋ก ์ค์ํ ํฌ๋งํด์ง์์ ๊ทธ ๋์์ ๋ํญ ํ๋ํ ๋ฐ ์๋ค. ํนํ ํ๋ฐ๊ธฐ ์ค์ํ ํฌ๋งํด์ง ๋์์๋ ๋ถ์ง์ ์ฅ ์ดํ ๋ชจ๋ ์ง๊ธ์ ๊ทผ์์ฐ์ 15๋ ์ด์ ๋ฐ 1983๋ ์ ์ด์ ์ถ์ ์ง์์ผ๋ก, ๋น์ ์์ผ์ด ์ง๋์ง ์์ ๋ง 39์ธ ์ง์๊น์ง ํฌํจ๋๋ค. ์ฌํด ํฌ๋งํด์ง ๋์์ ์ค 1986๋ ์์ ์์ผ์ด ์ง๋์ง ์์์ ๊ฒฝ์ฐ ๋ง 38์ธ์ ํด๋นํด ๊ทธ ๋ฒ์๋ ๊ฐ์๋ก ํ๋๋๋ ์ถ์ธ๋ค.
์ ํ์ํ์ ์ด๋ฒ ํฌ๋งํด์ง ์ ์ฒญ์ ์ทจํฉํด ๋ด๋ 1์ 2์ผ๊น์ง ํด์ง ์ ์ฐจ๋ฅผ ๋ง๋ฌด๋ฆฌํ ๊ณํ์ด๋ค. ์ ํ์ํ ๊ด๊ณ์๋ โ๊ณ ์ฐ๋ น, ๊ณ ์ฐ์ฐจ ์ง์๋ค์ โ์ 2์ ์ธ์โ ์ถ๋ฐ์ ๋๊ณ , ์ธ๋ ฅ ํจ์จํ๋ฅผ ํตํด ์ ๊ท ์ฑ์ฉ ์ฌ๋ ฅ์ ํ๋ํ๋ ์ฐจ์์์ ํฌ๋งํด์ง์ ์ํํ๊ฒ ๋๋คโ๊ณ ๊ทธ ๋ฐฐ๊ฒฝ์ ์ค๋ช ํ๋ค.
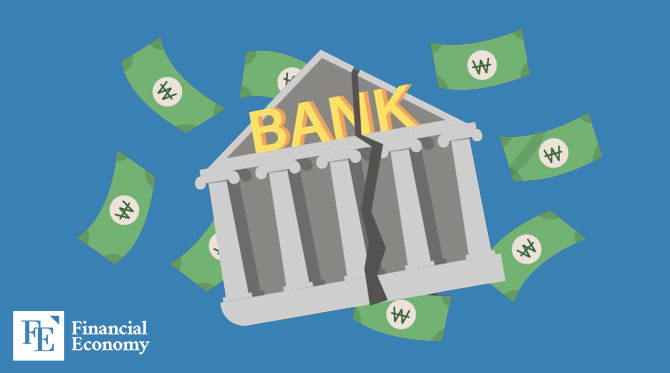
โ๋ ๋ฐ์ผ๋ ค๋โ ์ง์๋ค, โ๋ ์ฃผ๋ ค๋โ ์ํ๋ค
์ํ๊ถ์ ํฌ๋งํด์ง ์์ฆ์ด ๋๋ํ๋ฉด์ ๋์์๋ค์ ์ต์ ์ ํ์ด๋ฐ๊ณผ ์กฐ๊ฑด ๋ฑ์ ์ ์ธ์งํ๋ ๋ชจ์ต์ด๋ค. ์ํ์ด ์ ์ํ ์กฐ๊ฑด์ด ๊ธฐ๋์ ๋ชป ๋ฏธ์น๋ค๋ ์์ฌ์์ ๋ด๋น์น๋ฉด์๋, ๊ฒฝ๊ธฐ ๋ถํฉ์ ์ ๊ตญ ํผ๋๊น์ง ๊ฒน์น๋ฉด์ ์ถํ ์ํฉ์ด ๋ ์ ํํ ๊ฐ๋ฅ์ฑ์ ๋ฐฐ์ ํ ์ ์๊ธฐ ๋๋ฌธ์ด๋ค. ๋ ์ข์ ์กฐ๊ฑด์ ๊ธฐ๋ค๋ฆฌ๋ค ์คํ๋ ค ๋ ๊ฐ๋ฒผ์์ง ๋ดํฌ๋ฅผ ๋ฐ๊ณ ๋๊ฐ ์๋ ์๋ค๋ ๊ฒ ์ํ๊ถ์์ ํ๋ฌ๋์ค๋ ๋์ ์์ธ ์ด์ผ๊ธฐ๋ค.
์ค์ ๋ก ์ฌ ์๋ฐ๊ธฐ ์งํ๋ ์ํ๊ถ ํฌ๋งํด์ง์์๋ ์์ ๊ธ์ต์ ๊ฐ์กฐํ๋ ๊ธ์ต๋น๊ตญ์ ๋์น๋ฅผ ๋ณด๋ฉฐ ์ํ๋ค์ด ์ผ์ ํ ํน๋ณ ํด์ง๊ธ์ ์ถ์ํ๊ธฐ๋ ํ๋ค. ์ฌ๊ธฐ์ ๊ฐ์ข ๊ท์ ๋ก ์์ ํ๊ฒฝ์ด ์ ํํ๊ณ , ๋๋ด์ธ์ ์๊ธฐ ์ํฉ ๋ฆฌ์คํฌ๊ฐ ๊ณ ์ค๋ํ ์ํ๊ถ์ผ๋ก ์ ์ด ๋๋ฉด์ ์์ต์ฑ๊ณผ ์ฑ์ฅ์ฑ์ ๋ํ ๋ถ์ ๋ํ ํ๋๋ ์ํฉ์ด๋ค.
ํ ์์ค์ํ ๊ด๊ณ์๋ โ์ต๊ทผ ์ํ๊ถ ๊ณ ์ก ์ฐ๋ด์ ๋ํ ์ฌ๋ก ์ด ์ข์ง ์์ ๊ฒ ์ฌ์คโ์ด๋ผ๊ณ ์ง์ผ๋ฉฐ โ์ด๋ฐ ์ํฉ์์ ๊ณ์ ์ผํฌ๋ก IMFยท๊ธ์ต์๊ธฐ ์ฌํ ์ฐ๋ ค๊น์ง ์ ๊ธฐ๋๋ฉด์ ์์ผ๋ก ํฌ๋งํด์ง ๋ณด์์ ๋ ์ค์ด๋ค๋ฉด ์ค์ด๋ค์์ง, ๋์์ง ๊ฒ ๊ฐ์ง ์๋ค๋ ์ ๋ง์ด ์ง๋ฐฐ์ ์ด๋คโ๊ณ ์ํ๊ถ ๋ถ์๊ธฐ๋ฅผ ์ ํ๋ค.
๋น๋๋ฉด ์ ๋ฌด ๋๋ฉฐ ์ค ๊ณณ ์์ด
๊ฐ์๋ก ์ค์ด๋๋ ์คํ๋ผ์ธ ์์ ์ ๋ ๋ ๋ง์ ์ง์์ ์ํ ๋ฐ์ผ๋ก ๋ด๋ชจ๋ ์์๋ค. ์ํ์ฐํฉํ ์๋ฃ์ ์ํ๋ฉด ๊ตญ๋ด 6๊ฐ ์์ค์ํ(๊ตญ๋ฏผยท์ ํยทํ๋ยท์ฐ๋ฆฌยทSC์ ์ผยท์จํฐ์ํ) ์ ํฌ ์๋ 2012๋ ๋ง 4,729๊ฐ์์ ์ง๋ 3์ ๋ง 2,989๊ฐ๋ก 11๋ ์ฌ ์ 1,740๊ฐ(37%)๊ฐ ์ค์๋ค. ์์ ์ 10๊ณณ ์ค 4๊ณณ์ ์ฌ๋ผ์ง ์ ์ด๋ค. ์ฌํด 1๋ถ๊ธฐ๋ง ๋ผ์ด๋๊ณ ๋ณด๋ฉด ์์ค์ํ ์์ ์ 32๊ฐ๊ฐ ์์ด์ง๋ ๋์, ์ ์ค๋ ์์ ์ ์ 10๊ณณ์ ๊ทธ์ณค๋ค. ํ์ ์ฌ์ ๋ ์์ ๊ถ ์ค๋ณต, ๊ถ์ญ๋ณ ์คยท๋ํํ, ๊ทผ์ ์ ํฌ์ ํตํฉ์ด ๋๋ถ๋ถ์ด๋ค.
์์ ์ ์ถ์์ ๋ฐ๋ผ ํ์ ์ธ๋ ฅ๋ ๋ํญ ๊ฐ์ํ๋ค. ์์ค์ํ ์์ง์ ์๋ 3์ ๋ง ๊ธฐ์ค 6๋ง2,023๋ช ์ผ๋ก 2013๋ ๋ง ๋๋น 1๋ง4,488๋ช (19%) ๊ฐ์ํ๋ค. ์์ง์ ์๊ฐ ๊ฐ์ฅ ๋ง์ด ์ค์ด๋ ๊ณณ์ ํ๋์ํ์ผ๋ก 2021๋ 6,161๋ง1,302๋ช ์์ ์ง๋ํด 1๋ง686๋ช ์ผ๋ก 616๋ช (5.5%) ๊ฐ์ํ์ผ๋ฉฐ, ์ด์ด ์ฐ๋ฆฌ์ํ(380๋ช ยท2.8%), ์ ํ์ํ(123๋ช ยท1.0%), ๊ตญ๋ฏผ์ํ(80๋ช ยท0.5%) ๋ฑ ์์ ๋ณด์๋ค.
์ด์ฒ๋ผ ์์ค์ํ์ ์์ ์ ์์ ์์ง์ ์๊ฐ ์ผ์ ํ ๊ฐ์ํ ๋ฐฐ๊ฒฝ์ผ๋ก๋ ํฌ๋ฐ๋ฏน ์ดํ ํ์ฑํํ ๋น๋๋ฉด ๊ธ์ต์ด ๊ผฝํ๋ค. ๊ธฐ์กด ์์ ์ ์์ ์ฒ๋ฆฌํ๋ ์ ๋ฌด์ ๋๋ถ๋ถ์ ์จ๋ผ์ธ์ผ๋ก ์ฒ๋ฆฌํ ์ ์๊ฒ ๋๋ฉด์ ์ํ๋ค์ด ๋น์ฉ ์ ๊ฐ์ ์ํด ์์ ์ ์ถ์์ ๋์ ๊ฒ์ด๋ค. ํ๊ตญ์ํ์ ๋ฐ๋ฅด๋ฉด ์ง๋ํด ๋ง ๊ตญ๋ด์ํ์ ์ธํฐ๋ท๋ฑ ํน ๋ฑ๋ก ๊ณ ๊ฐ ์๋ 2์ต704๋ง ๋ช ์ผ๋ก 1๋ ์ ๊ณผ ๋น๊ตํด 8.5% ๋์๋ค.